Making large model training more efficient, Singular Mole defines the next generation of AI computing with connected innovation solutions
Source: Strange Mole Author: Strange Mole
Recently, the DeepSeek phenomenon has triggered the industry's thinking and controversy over the construction of large-scale data centers. At the training end, DeepSeek uses open source models to reduce training costs through algorithm optimization (such as sparse computing and dynamic architecture), so that enterprises can realize the training of high-performance AI large models at a low cost. On the inference side, DeepSeek accelerates the migration of AI applications from training to the inference stage. Therefore, there are views that the demand for computing power will slow down after DeepSeek. However, more domestic and foreign institutions and research reports believe that DeepSeek reduces the threshold of AI applications, will accelerate the application of AI large model, attract more enterprises to enter the track, computing power demand will continue to grow, but the focus of demand from "single card peak performance" to "cluster energy efficiency optimization". For example, SemiAnalysis predicts that global data center capacity will grow from 49GW in 2023 to 96GW in 2026, with new smart computing center capacity accounting for 85% of the increase. Recently, the world's four giants (Meta, Amazon, Microsoft and) announced a total of more than $300 billion in AI infrastructure spending in 2025, an increase of 30% compared to 2024.
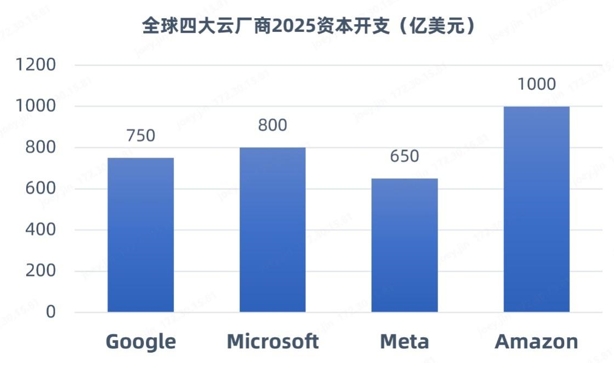
(Data source: Tech giant public Disclosure Report)
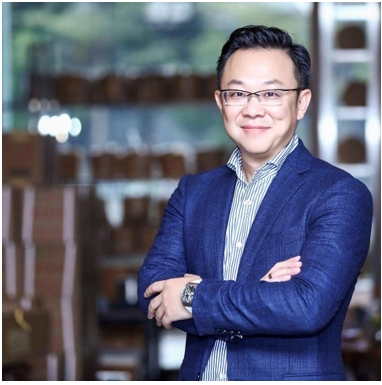
(Photo: Tian Muchen, founder and CEO of Strange Moore)
"Scaling Law is here to stay," said Michen Tian, founder and CEO of Kiwi Moore. From Transformer's dominance to the innovation breakthrough of MoE's expert model, the AI field is moving toward a trillion or even ten trillion parameter scale of AI large model training era. The advent of DeepSeek-R1 inference model is inseparable from the huge training accumulation of the basic model Deepseek-V3. In this context, powerful computing power clusters are still the cornerstone of supporting AI. How to improve the linear acceleration ratio of cluster has always been the core topic of the industry. At the same time, the importance of AI computing power network is becoming increasingly prominent, which allows data to be quickly transmitted at all levels and dimensions of the cluster, and realizes the efficient mobilization of node resources." To this end, as the industry's leading provider of AI network full-stack interconnection products and solutions, Chimol has given a set of highly competitive solutions based on high-performance RDMA and Chiplet technology, using the three concepts of "Scale Out", "Scale Up" and "Scale Inside". Improve the transmission efficiency of computing infrastructure between networks, between chips and within chips, enabling the development of intelligent computing power.
Scale Out - Break the system transmission bottleneck
DeepSeek's success proves that open source models have certain advantages over closed source models, and with the evolution of intelligent models, the increase of model size will still be one of the main trends in the development of the industry. In order to complete the training task of a large AI model with a scale of 100 billion and trillion parameters, the common approach is to adopt Tensor parallel (TP), Pipeline parallel (PP), and Data parallel (DP) strategies to split the training task. With the advent of the MoE (Mixture of Experts) model, in addition to the aforementioned parallel strategies, expert parallelism (EP) has also been introduced. Among them, EP and TP communication data overhead is large, which is mainly dealt with by Scale Up interconnection. The communication cost of DP and PP parallel computing is relatively small, which is mainly dealt with by Scale Out interconnection.
Therefore, as shown in the following figure, there are two types of interconnection domains in the current mainstream Wanka cluster: GPU southbound Scale Up Domain (SUD) and GPU northbound Scale Out Domain (SOD). Tian Mochen stressed: "To build a large-scale and efficient intelligent computing cluster in the way of Scale Up and Scale Out is an effective means to deal with the outbreak of computing power demand."
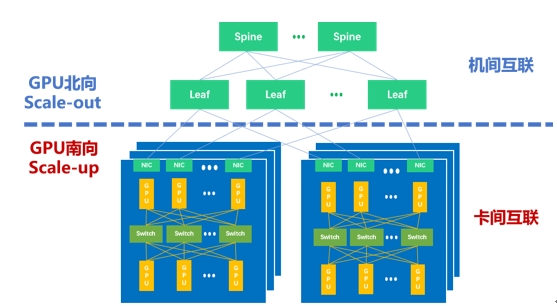
Calculate the Scale Up and Scale Out of the cluster
In this cluster network, Scale Out focuses on horizontal/horizontal scaling, emphasizing the expansion of the cluster size by adding more compute nodes. At present, remote Direct memory access (RDMA) has become the mainstream choice for building Scale Out networks. As a host-offload/host-bypass technology, RDMA provides direct access from the memory of one computer to the memory of another computer with low latency and high bandwidth, and plays an important role in large-scale clusters. As shown in the figure below, RDMA mainly consists of InfiniBand (IB), Ethernet-based RoCE, and TCP/ IP-based iWARP. Among them, IB and Ethernet RDMA are the most widely used technologies in computing clusters.
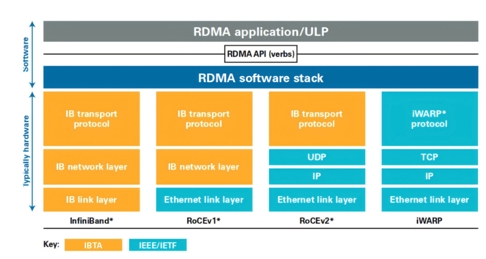
RDMA application and implementation (Source: Zhihu@savir)
IB is a network communication technology specially developed for RDMA, which has the advantages of high bandwidth and low latency. IB is a lossless network by default, and no special Settings are required. Thanks to these advantages, IB has dominated the Scale Out network construction in the past. However, IB requires network cards and switches that specifically support the technology at 5-10 times the price of traditional networks, with relatively high costs and long lead times for IB switches. At the same time, IB compatibility is poor, and it is difficult to be compatible with most Ethernet devices, such as network cards, cables, switches, and routers, and cannot become a unified development route in the industry. With the increase of cluster scale, Ethernet RDMA has been widely supported by mainstream vendors. Ethernet RDMA also has the advantages of high speed, high bandwidth and low CPU load, and has been equal to IB performance in terms of low latency and lossless network characteristics. At the same time, Ethernet RDMA has better openness, compatibility and unity, which is more conducive to large-scale networking cluster. From some industry representative cases, such as Bytedance's 10,000 card cluster, Meta's tens of thousands of card cluster, and Tesla's hope to build a 100,000 card cluster, all unanimously choose the Ethernet solution. In addition, because the hardware is common and the operation and maintenance is simple, the Ethernet RDMA scheme is more cost-effective. Although Ethernet RDMA has been recognized as the general trend of Scale Out in the future, Tian Mochen pointed out: "If it is based on RoCEv2, there are still some problems, such as out-of-order retransmission, load sharing is not perfect, there is a Go-back-N problem, and DCQCN deployment and tuning is complex." In 10,000-card and 10,000-card clusters, the industry needs enhanced Ethernet RDMA to address these challenges, and Ultra Ethernet Transport (UET) is a key technology in the next generation of AI computing and HPC." In order to further realize the potential of Ethernet and RDMA technology, Broadcom, Cisco, Arista, Microsoft, Meta and other companies have led the formation of the Ultra Ethernet Alliance (UEC). As shown in the following figure, in the preview version of UEC specification 1.0, UEC has comprehensively optimized the Transport Layer from the aspects of software API, transport layer, link layer, network security and congestion control. Key functions include FEC (forward error correction) statistics, link layer retransmission (LLR), multipath packet eruption, next-generation congestion control, flexible sorting, end-to-end telemetry, switch offloading, and more. According to AMD, UEC-ready systems can provide five to six times higher performance than traditional RoCEv2 systems.
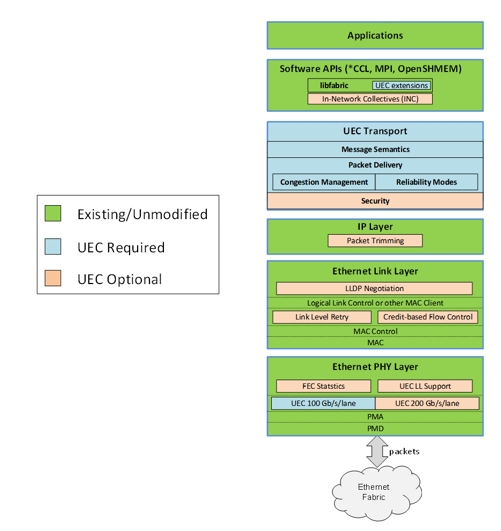
UEC Specification 1.0 schematic (Source: UEC)
Tian said: "UEC is an international alliance specifically established to Scale Out the interconnection of AI networks and is committed to optimizing AI and HPC workloads with Modernized RDMA. With the key capabilities of UEC, Scale Out networks are able to take full advantage of all available transmission paths within the system and minimize network congestion. "Today's RDMA ROCE-based solutions can also upgrade their Ethernet offerings in the future by implementing the UEC standards to create lossless trunking communications on a larger scale." Kiwi NDSA-SNIC AI native intelligent network card created by Kiwi Moore is a UEC-ready solution with performance comparable to the global benchmark ASIC products. Kiwi NDSA SmartNIC provides industry-leading performance, supports transmission bandwidth up to 800Gbps, provides data transmission latency down to μs, meets the current data center industry upgrade requirements of 400Gbps-800Gbps, and can realize lossless data transmission between terabytes of 10 million card clusters.
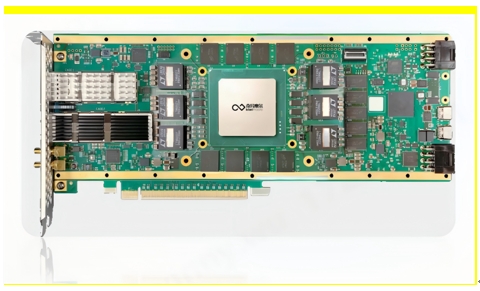
Kiwi NDSA-SNIC AI native intelligent network Card solution (Source: Kiwi Moore)
With key features such as path-aware congestion control, ordered messaging, selective acknowledgement retransmission, adaptive routing, and packet spraying in UEC-ready RDMA, Kiwi NDSA-SNIC can fully guarantee the stable transmission of data between AI networks. For example, the adaptive routing and packet spraying functions provided by Kiwi NDSA-SNIC can give full play to the performance of high-speed networks, support advanced packet spraying, provide multi-path packet delivery and fine-grained load balancing, and effectively deal with transport congestion. Similar use cases include In-Order Message Delivery to reduce system latency, Path Aware Congestion Control to optimize multi-path packet flow, and so on. In addition, Kiwi NDSA-SNIC has many other key features. For example, Kiwi NDSA-SNIC has excellent concurrency, supporting up to millions of queue pairs and expandable memory space up to GB. Kiwi NDSA-SNIC is programmable for a variety of network task acceleration, bringing continuous innovation to Scale Out networks and guaranteeing seamless compatibility with future industry standards. In summary, Kiwi NDSA-SNIC AI native intelligent network card is a high-performance, programmable Scale Out network engine that will open a new chapter in the development of AI network Scale Out. Tian Muchen said: "At present, Strange Moore has become a member of the UEC alliance. With the gradual transition of Ethernet to super-Ethernet, Chimol is willing to join hands with alliance partners to discuss and practice the formulation and improvement of Scale Out standards, and bring leading performance UEC solutions to the industry in the first time to promote the development of AI network Scale Out technology."
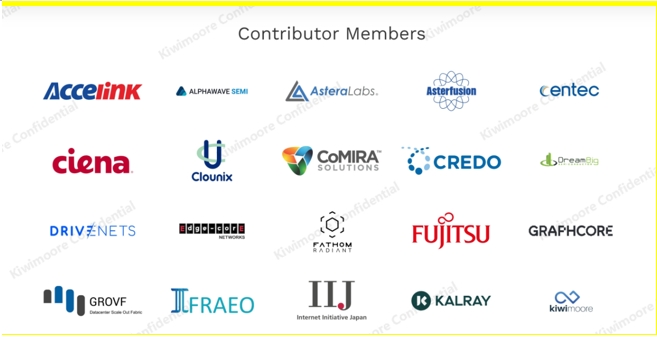
Strange Moore UEC Member (Source: UEC official website)
Scale Up - Make computing chips work together more efficiently
Different from horizontal/horizontal Scale Out, Scale Up is vertical/upward scale, with the goal of creating hypernodes with high bandwidth interconnection within the machine. As mentioned above, TP tensor parallelism and EP expert parallelism require higher bandwidth and lower time delay for global synchronization. By means of Scale Up, more computing power chip Gpus are concentrated on one node, which is a very effective way to cope. Today's Scale Up is actually an on-machine GPU-GPU networking mode with ultra-high Bandwidth as the core, and a name is HBD (High Bandwidth Domain).
The launch of Nvidia GB200 NVL72 leads the extensive discussion of HBD technology in the AI network ecology at home and abroad. The Nvidia GB200NVL72 server is a typical super-large HBD, enabling ultra-high bandwidth interconnection between 36 GB200 groups (36 Grace cpus, 72 B200 Gpus). In this HBD system, the fifth-generation NVLink is the most critical, it can provide GPU-GPU two-way 1.8TB transmission rate, so that this HBD system can be used as a large GPU, training efficiency compared to the H100 system increased by 4 times, energy efficiency increased by 25 times.
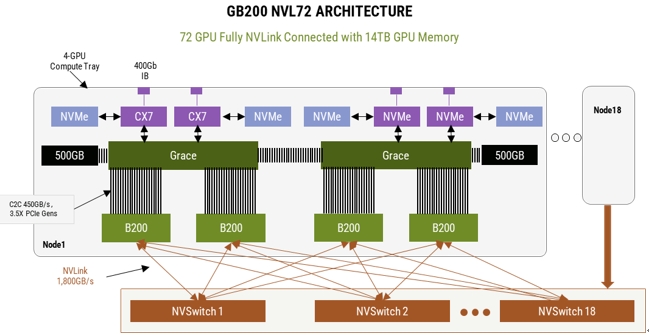
NVL72 Connected Architecture (Source: Nvidia)
Like IB, NVLink is also dominated by Nvidia, although the performance is strong but the ecology is closed, and only serves Nvidia's high-end GPU. Due to the absence of technologies such as NVLink and NVSwitch, other manufacturers previously mainly used full mesh or cube-mesh structure, with 8-card interconnection, and 16-32 card interconnection is the next generation solution. The DeepSeek incident has triggered different industry expectations for the above-mentioned NVLink and HBD demand. However, in the medium and long term, compared with the software iteration speed calculated in hours, the hardware iteration is a gradual process calculated in years, and will not be achieved overnight. According to SemiAnalysis, the standard for large models will only continue to rise with future model releases, but in terms of economic utility, the corresponding hardware must be used and effective for 4-6 years, not just until the next model is released. In this regard, Tian Muchen believes: "There is uncertainty in the future MoE model's advanced route to a certain extent, and innovation can happen at any time." However, the ecological closed loop of domestic AI network is imperative. Nvidia NVLink and Cuda moat still exist, first to solve the problem of Scale Up domestic alternatives, and then to see what extent. In the future, with the coordinated development of hardware and software ecology such as domestic large model and chip architecture, it is expected to gradually realize the closed-loop of domestic computing power." Today, tech giants are uniting the ecosystem upstream and downstream in terms of efficient GPU-GPU interconnection mainly divided into two schools: memory semantics and message semantics. Memory semantics Load/Store/Atomic is the native semantics of GPU internal bus transmission, Nvidia NVLink is based on memory semantics, NVLink UAlink is also based on this semantics; Message semantics uses DMA semantics similar to Scale Out, Send/Read/Write, to package and transmit data. Companies such as Amazon and Tenstorrent build Scale Up interconnection schemes based on message semantics. Memory semantics and message semantics are different. Memory semantics is the native semantics of GPU internal transmission, which has less processor burden and higher efficiency in terms of packet volume. Message semantics use the way of data packaging, as the size of the packet increases, the performance gradually catches up with the memory semantics, and as the size of the AI large model increases, this is also very important. However, Tian Mochen pointed out: "Whether it is memory semantics or message semantics, for manufacturers, are facing some common challenges, such as the traditional GPU straight out IO integration in the GPU, performance improvement is strictly limited by the size of the light mask, leaving IO space is very limited, IO density improvement is difficult; Scale Up network and data transmission protocol are complex, and most computing chip manufacturers lack relevant experience, especially in the development of switch chips. In addition to NVLink, other Scale Up protocols are not mature and uniform, and protocol iteration has caused a huge headache for computing chip iteration."GPU IO integrated inside the GPU (Source: Singular Mole)
In order to better meet the above challenges, the industry has proposed an innovative GPU direct out of the way - computing and IO separation. The Singular Mole NDSA-G2G interconnection scheme is a very competitive scheme in this technical path. With NDSA-G2G, computing cores and IO cores can be decoupled and interconnected through the universal core interconnection technology UCIe. The advantage of this is that with only a small sacrifice of chip area (a few percent), nearly 100% of the valuable intermediary layer resources can be used for computing, and the number of IO cores can be flexibly increased according to customer needs, and the compute cores and IO cores can be based on different process technologies. In addition, the multiplexing characteristics of IO cores can significantly improve the performance and cost performance of high-performance computing chips. The second major advantage of NDSA-G2G is improved IO density and performance, with high bandwidth, low latency and high concurrency characteristics. In terms of high bandwidth, based on NDSA-G2G cores, 1TB network layer throughput and TB GPU side throughput can be achieved. In terms of low latency, NDSA-G2G core provides hundred-Ns level data transmission delay and NS-level D2D data transmission delay. In terms of high concurrency, the product supports up to millions of queue pairs, extending the memory resources in the system. In other words, with the help of the Kimol NDSA-G2G core, the domestic AI chip can achieve independent breakthrough and build a Scale Up solution with performance comparable to Nvidia NVSwitch+NVLink.
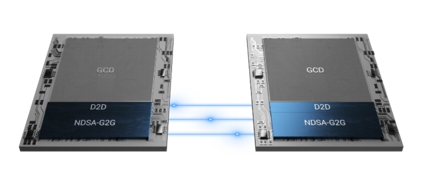
Kiwi NDSA-G2G product schematic (Source: Kimol)
The third advantage of the NDSA-G2G is its flexibility. As mentioned above, the current Scale Up technology route is not uniform, and most of the intelligent computing center manufacturers use their own protocols or alliance agreements dominated by themselves. This leads to high performance computing chips need to be designed to consider the next 2 to 3 years, or even 3 to 5 years of protocol development, which has a very big challenge. NDSA-G2G allows flexible upgrade of IO cores by separating computing cores and IO cores, while NASG-G2G is programmable and can support various IO protocols currently on the market. This flexibility allows HPC chip manufacturers to cope with the challenges of the current Scale Up technology route is not uniform and the protocol is confusing. At the same time, Tian Muichen also called for: "I hope that the science and technology industry can embrace an open and unified physical interface in the direction of Scale Up, to achieve better collaborative development, which is also a key step in creating a domestic autonomous controllable computing base."
Scale Inside - Comprehensively improve the transmission efficiency of computing chips In the process of rapid development of Scale Out and Scale Up, as a basic unit of computing power, the progress of Scale Inside has not fallen, and is committed to making up for the slowing down of Moore's Law through advanced packaging technology. In the entire intelligent computing system, computing chips with higher computing power can further improve the performance level of Scale Up and Scale Out, making the training of large AI models more efficient. At present, the cost of a single high-performance computing chip is already very terrible, and with the further development of the process, this figure will continue to soar, so Chiplet technology has been widely valued. Chiplet technology allows high performance computing chips to be built through hybrid packaging, which means that the computing unit and other functional units such as IO and storage can be implemented in different processes, with high flexibility, allowing manufacturers to customize the core according to their needs, not only significantly reducing the cost of chip design and manufacturing, but also reducing the cost of chip design and manufacturing. The yield can also be greatly improved. In the direction of Scale Inside, Kiwi Mole can provide a wealth of Chiplet technology solutions, including Kiwi Link UCIe Die2Die interface IP, Central IO Die,3D Base Die series, etc. Kiwi Link supports the UCIe standard with industry-leading high bandwidth, low power, low latency features and supports a variety of package types. Kiwi Link supports transmission rates of up to 16 to 32 GT/s and transmission latency as low as ns, and supports Multi-Protocol, including PCIe, CXL, and Streaming.
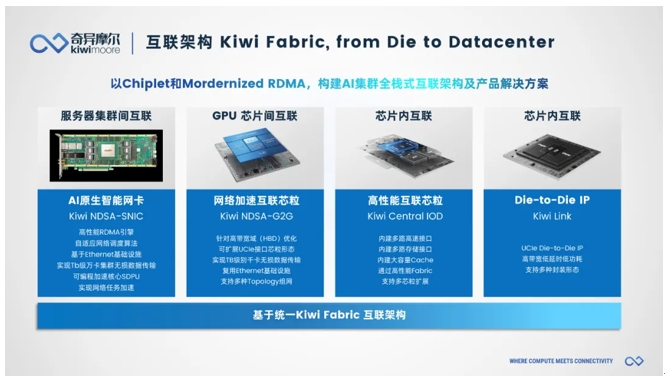
Kiwi Fabric Connectivity Architecture (Source: Kiwi Mole)
In summary, the solution of Strange Mole can promote the improvement of the training efficiency of AI large models from the three perspectives of "Scale Out", "Scale Up" and "Scale Inside". In terms of Scale Out, Singular Mole has been a member of the ultra-Ethernet Alliance UEC, and can respond to UEC specification 1.0 and subsequent specifications in the first time. In terms of Scale Up, Chimol NDSA-G2G cores can not only help technology companies create Scale Up solutions with performance comparable to Nvidia NVSwitch+NVLink, adapt to various technical routes and protocols, but also lead the design innovation of computing chips. In the Scale Inside solution, Kiwi Link UCIe Die2Die interface IP, Central IO Die, 3D Base Die series and other solutions can help manufacturers create high-performance computing chips with efficient transmission capabilities. These solutions well fulfill the mission of Chiplet and RDMA technology to build the cornerstone of AI high-performance computing. "For the domestic AI large model and the domestic AI chip industry, Strange Mole's solution is a representative of new quality productivity, and it has greater potential worth tapping." In order to realize the "Chinese dream" of the domestic AI chip industry, Chimol not only provides IO cores supporting the most cutting-edge protocols to achieve high speed, high bandwidth, and low latency transmission performance, but also takes a unique path on the Chiplet route to help create higher performance AI chips with innovative chip architecture. Chimol is willing to work with domestic companies to contribute to the development of the domestic AI chip industry and jointly sketch a broad blueprint for the development of domestic AI." Tian Momochen finally said.
免责声明: 本文章转自其它平台,并不代表本站观点及立场。若有侵权或异议,请联系我们删除。谢谢! Disclaimer: This article is reproduced from other platforms and does not represent the views or positions of this website. If there is any infringement or objection, please contact us to delete it. thank you! 矽源特科技ChipSourceTek |